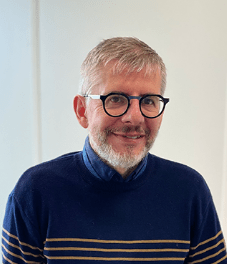
Multilevel Physics Informed Methods
Serge Gratton is a Professor of Exceptional Class in Applied Mathematics at INP-IRIT. He has published over a hundred articles in leading international journals, particularly in the fields of data assimilation and numerical optimization for machine learning. He has co-supervised 25 doctoral students and is an associate editor for two leading international journals in optimization (SIOPT and OMS). He heads two AI programs: a specialized Master’s in AI and a dual-degree engineering program with INSA-ENSEEIHT. Since 2019, he has held a chair in the field of machine learning under physical constraints, within which he has developed recurrent neural structures suited to the temporal prediction of the trajectories of partially observed chaotic dynamic systems. His involvement in ANITI has steadily increased: as a chair holder, academic leader, deputy scientific director, and now as the scientific director.
The approximation of the solution of partial differential equations (PDEs) using artificial neural networks (ANNs) dates back to the 90s. However, it is only in recent years that this topic has emerged as an active field of research. Since their introduction, Physics-Informed Neural Networks (PINNs) have garnered increasing interest in this field. Their effectiveness in practice has been supported by theoretical results. However, training these networks remains challenging, particularly due to slow convergence when approximating solutions characterized by high frequencies. To improve this situation, we introduce two distinct approaches. Firstly, the ‘deep-ddm’ method, which combines domain decomposition methods (DDM) with an innovative coarse space correction. Secondly, a multi-level algorithm designed for nonlinear optimization in PINNs, employing specialized neural architectures for enhanced performance. Both methods demonstrate significant improvements in solving PDEs. The deep-ddm method offers accelerated convergence and efficient information exchange between subdomains. Meanwhile, the multi-level algorithm yields superior solutions and computational savings. This synergy of classical and contemporary methods opens new avenues in applying PINNs to complex PDEs.
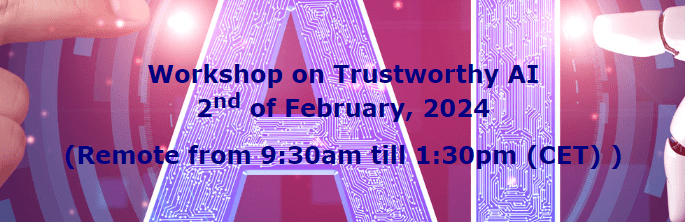